At Professor Xiayu Xu’s invitation, Assistant professor Ziyue Xu from the National
Institutes of Health, visited BEBC on 21st Nov, 2017 and delivered a report
entitled “Recent Advances of Deep Learning Methods for Pulmonary Image
Analysis with Incomplete Training Labels” in the 308 meeting room in the School of Life Science
and Technology.
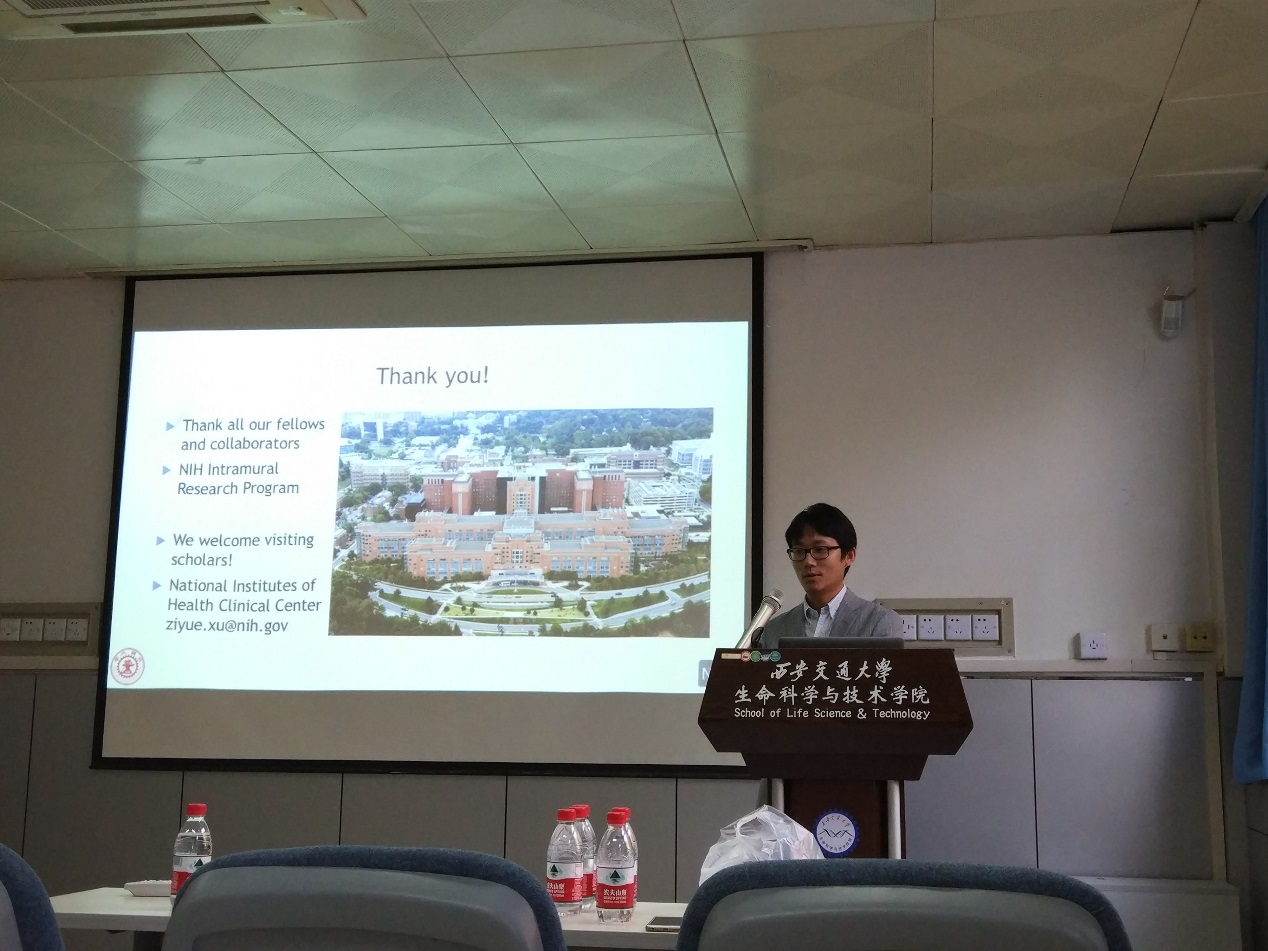
His report showed
that computer vision and image analysis is among the fields that adopted deep
learning most successfully. Such success is enabled by computational power,
learning algorithm, and data availability. For medical image analysis, however,
data and label pose a major challenge. As compared with millions of
well-annotated natural images, we usually only have at most a few thousand for
medical tasks. Among them, majority has no annotation, few with labels, and
fewer of high quality. In this work, we discuss the challenges and possible
solutions in applying deep learning methods for pulmonary image analysis when
we do not have well-annotated training data. Common tasks are covered including
pathological lung segmentation, lobe fissure estimation, airway extraction, and
disease pattern classification. For each candidate task, based on its unique
features, we present the reason behind the choice of a specific deep learning
structure. Further, we discuss the characteristics of pulmonary images, and how
to design and generate the training data that is usually constraint by limited
clinical resources. Based on the result, we show that for certain tasks in medical
image analysis, incomplete label is feasible for training if it sufficiently
covers the variance of target subject. In the end of the report, he had a
heated discussion with teachers and students in BEBC.